Are there any useful variables that you can engineer with the given data?
Review a list of the feature names below, from which we can engineer:
- The total number of dependents in the home (‘Dependents’) can be engineered from the sum of ‘Kidhome’ and ‘Teenhome’
- The year of becoming a customer (‘Year_Customer’) can be engineered from ‘Dt_Customer’
- The total amount spent (‘TotalMnt’) can be engineered from the sum of all features containing the keyword ‘Mnt’
- The total purchases (‘TotalPurchases’) can be engineered from the sum of all features containing the keyword ‘Purchases’
- The total number of campaigns accepted (‘TotalCampaignsAcc’) can be engineered from the sum of all features containing the keywords ‘Cmp’ and ‘Response’ (the latest campaign)
Deriving Some useful Data
%scala
import spark.implicits._
import org.apache.spark.sql.functions._
spark.sql("set spark.sql.legacy.timeParserPolicy=LEGACY")
val derivedDF = Finalmarketingdataframe.select($"ID", $"Income", $"Kidhome" + $"Teenhome" as "Dependents", year(to_timestamp($"Dt_Customer", "MM/dd/yy")) as "Year_Customer", $"MntWines" + $"MntFruits" + $"MntMeatProducts" + $"MntFishProducts" + $"MntSweetProducts" + $"MntGoldProds" as "TotalMnt", $"NumDealsPurchases" + $"NumWebPurchases" + $"NumCatalogPurchases" + $"NumStorePurchases" as "TotalPurchases", $"AcceptedCmp1" + $"AcceptedCmp2" + $"AcceptedCmp3" + $"AcceptedCmp4" + $"AcceptedCmp5" as "TotalCampaignsAcc", $"Country")
import spark.implicits._
import org.apache.spark.sql.functions._
spark.sql("set spark.sql.legacy.timeParserPolicy=LEGACY")
val derivedDF = Finalmarketingdataframe.select($"ID", $"Income", $"Kidhome" + $"Teenhome" as "Dependents", year(to_timestamp($"Dt_Customer", "MM/dd/yy")) as "Year_Customer", $"MntWines" + $"MntFruits" + $"MntMeatProducts" + $"MntFishProducts" + $"MntSweetProducts" + $"MntGoldProds" as "TotalMnt", $"NumDealsPurchases" + $"NumWebPurchases" + $"NumCatalogPurchases" + $"NumStorePurchases" as "TotalPurchases", $"AcceptedCmp1" + $"AcceptedCmp2" + $"AcceptedCmp3" + $"AcceptedCmp4" + $"AcceptedCmp5" as "TotalCampaignsAcc", $"Country")
Display Derived Data
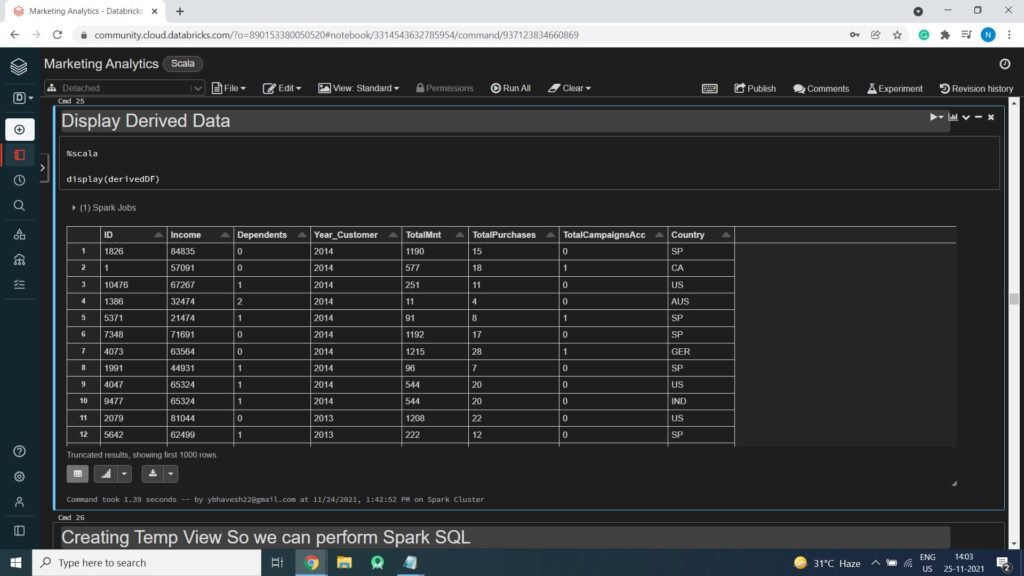
Creating Temp View So we can perform Spark SQL
%scala
derivedDF.createOrReplaceTempView("DerivedData");
derivedDF.createOrReplaceTempView("DerivedData");
Scatter Plot TotalMnt VS Income
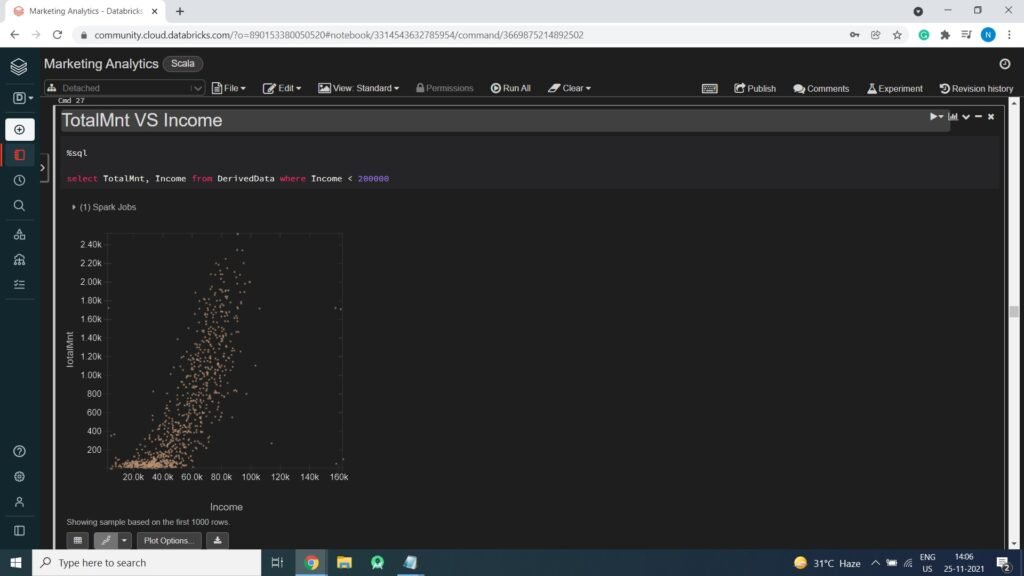
NumDealsPurchases VS Dependents

TotalCampaignsAcc VS Income

Dependents VS TotalCampaignsAcc
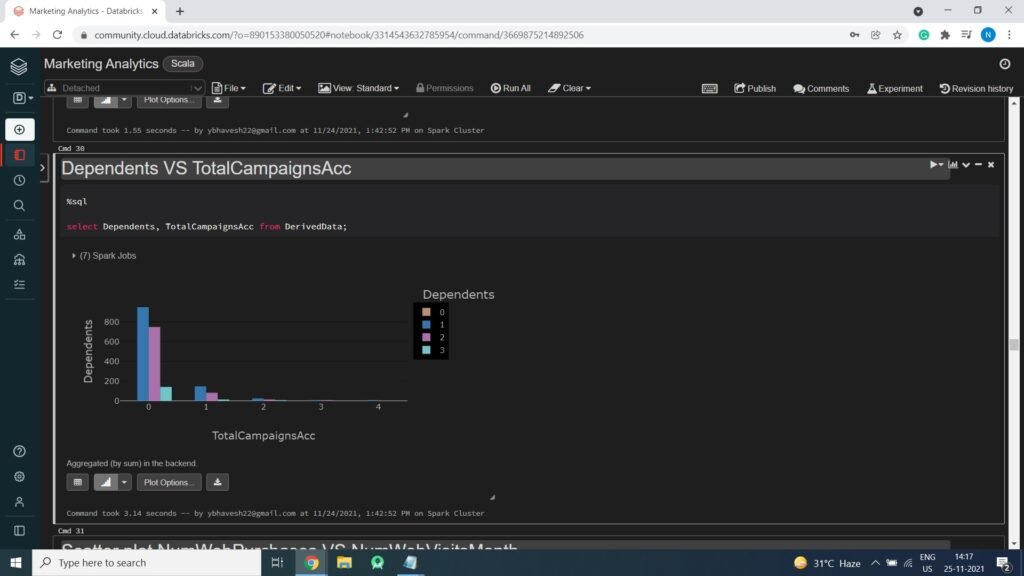
Scatter plot NumWebPurchases VS NumWebVisitsMonth

Scatter Plot NumDealsPurchases VS NumWebVisitsMonth

Section 02: Statistical Analysis
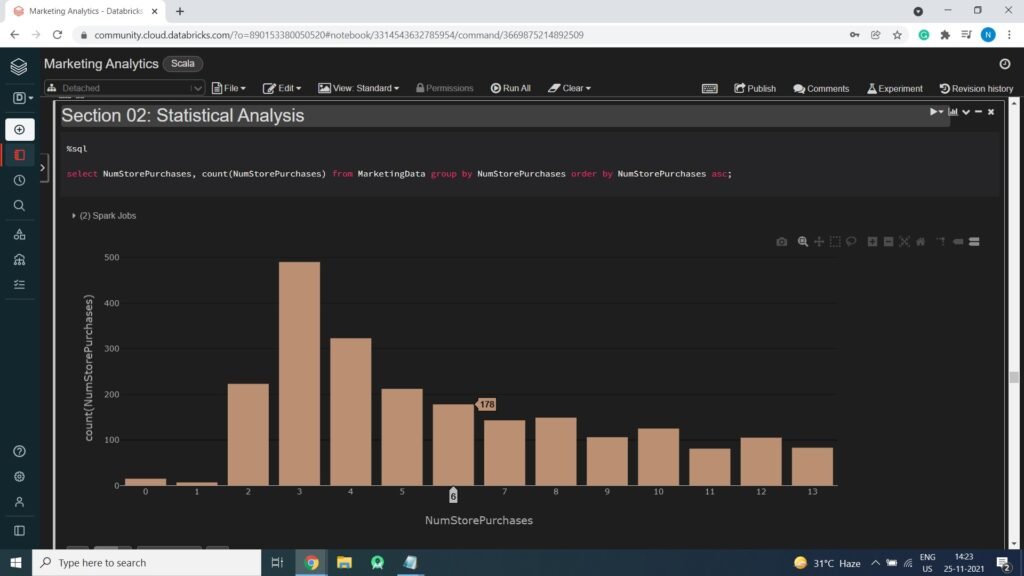
Total Number of Purchases by Country
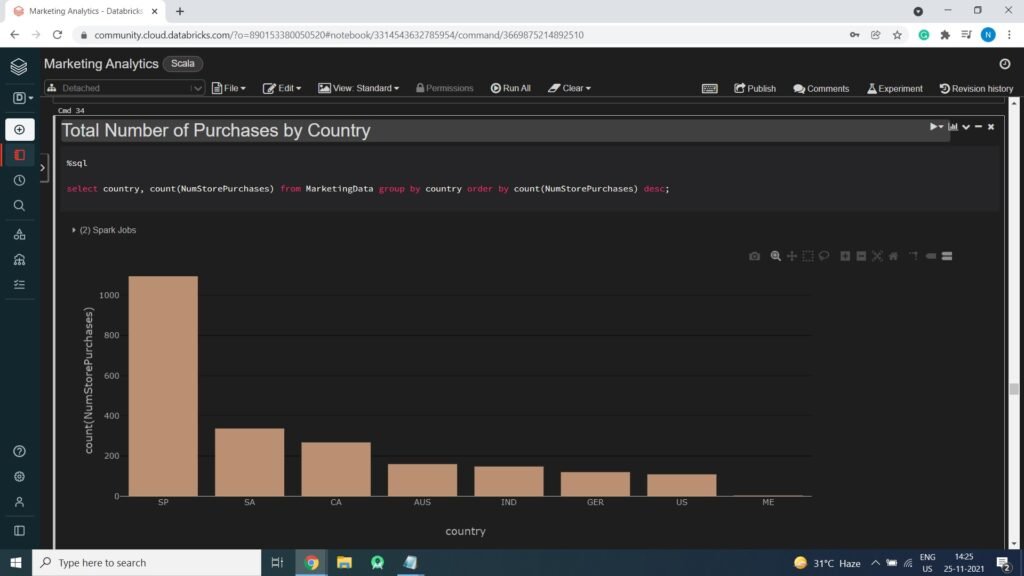
Total Amount Spent by Country
