Patient's Age Patient's Income Patient's Occupation All in One Scatter Plot Loading Data into DataFrame %scala // File location and type val file_location = "/FileStore/tables/First_Health_Camp_Attended.csv" val file_type = "csv" // CSV options val infer_schema = "true" val first_row_is_header = "true" val delimiter = "," // The applied options are for CSV files. For other file types, these will be ignored. val First_Health_Camp_Attended = spark.read.format(file_type) .option("inferSchema", infer_schema) .option("header", first_row_is_header) .option("sep", delimiter) .load(file_location) display(First_Health_Camp_Attended) Count of Data (Total Records) %scala First_Health_Camp_Attended.count() res12: Long = 6218 Displaying Statistics of Data %scala display(First_Health_Camp_Attended.describe()) Print Schema of Data %scala First_Health_Camp_Attended.printSchema() root |-- Patient_ID: integer (nullable…
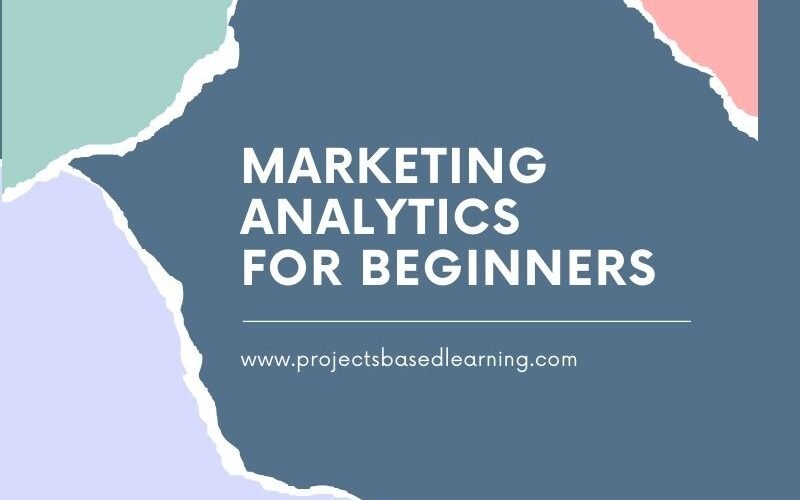
Marketing analytics consists of both qualitative and quantitative, structured and unstructured data used to drive strategic decisions in relation to brand and revenue outcomes. Overall goalYou're a marketing analyst and you've been told by the Chief Marketing Officer that recent marketing campaigns have not been as effective as they were expected to be. You need to analyze the data set to understand this problem and propose data-driven solutions.Section 01: Exploratory Data Analysis Are there any null values or outliers? How will you wrangle/handle them?Are there any variables that warrant transformations?Are there any useful variables that you can engineer with the given data?Do…
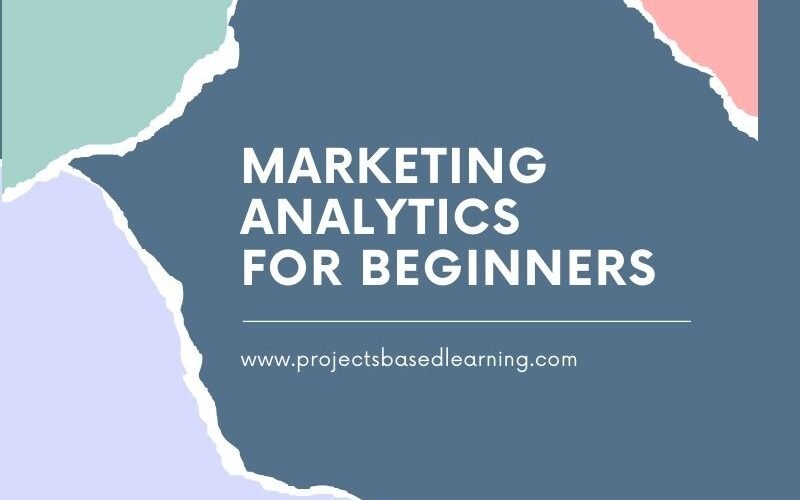
Are there any useful variables that you can engineer with the given data?Review a list of the feature names below, from which we can engineer:The total number of dependents in the home ('Dependents') can be engineered from the sum of 'Kidhome' and 'Teenhome'The year of becoming a customer ('Year_Customer') can be engineered from 'Dt_Customer'The total amount spent ('TotalMnt') can be engineered from the sum of all features containing the keyword 'Mnt'The total purchases ('TotalPurchases') can be engineered from the sum of all features containing the keyword 'Purchases' The total number of campaigns accepted ('TotalCampaignsAcc') can be engineered from the sum of…
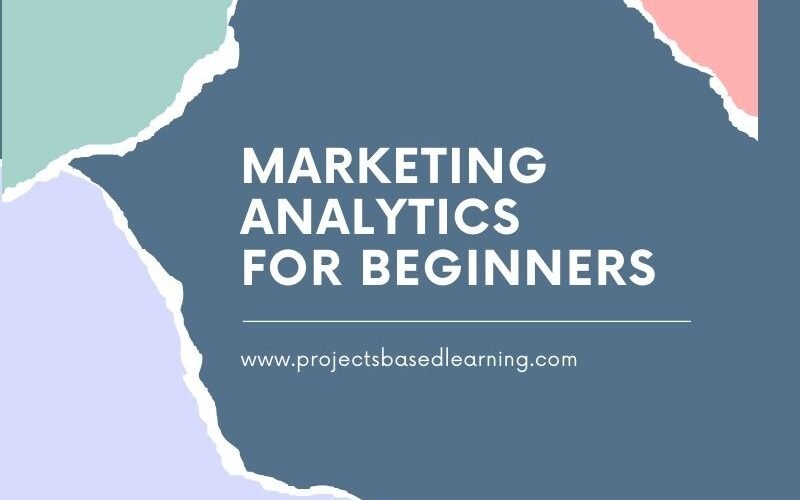
NumStorePurchases VS MntGoldProds MntFishProducts Distribution Campaign 1 Campaign 2 Campaign 3 Campaign 4 Campaign 5 Section 03: Data Visualization Products VS Amount Spent Purchases Conclusion Recall the overall goal: You're a marketing analyst and you've been told by the Chief Marketing Officer that recent marketing campaigns have not been as effective as they were expected to be. You need to analyze the data set to understand this problem and propose data-driven solutions...Summary of actionable findings to improve advertising campaign success:Advertising campaign acceptance is positively correlated with income and negatively correlated with having kids/teensSuggested action: Create two streams of targeted advertising campaigns,…
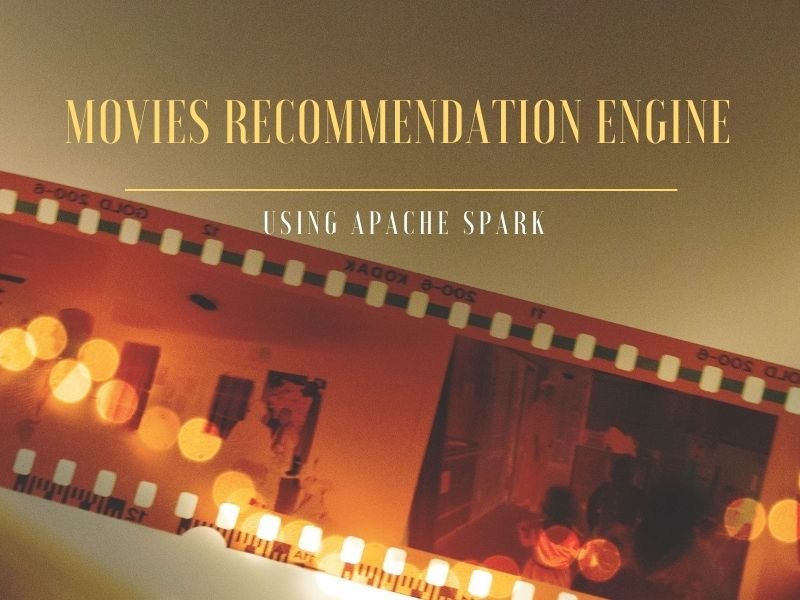
Movies are loved by everyone irrespective of age, gender, race, color, or geographical location. A recommendation system is a filtration program whose prime goal is to predict the “rating” or “preference” of a user towards a domain-specific item or item. Recommendation systems encompass a class of techniques and algorithms that can suggest “relevant” items to users. They predict future behavior based on past data through a multitude of techniques. Problem Statement or Business Problem In this project, we will generate top 10 movie recommendations for each user as well as generate top 10 user recommendations for each movie. Attribute Information…
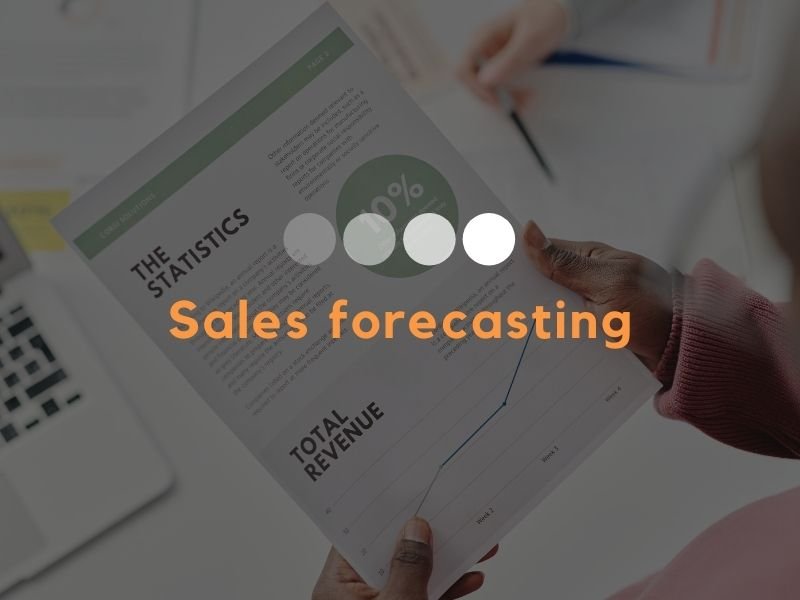
Sales forecasting is the process of estimating future sales. Accurate sales forecasts enable companies to make informed business decisions and predict short-term and long-term performance. Companies can base their forecasts on past sales data, industry-wide comparisons, and economic trends. It is easier for established companies to predict future sales based on years of past business data. Newly founded companies have to base their forecasts on less-verified information, such as market research and competitive intelligence to forecast their future business. Sales forecasting gives insight into how a company should manage its workforce, cash flow, and resources. In addition to helping a…
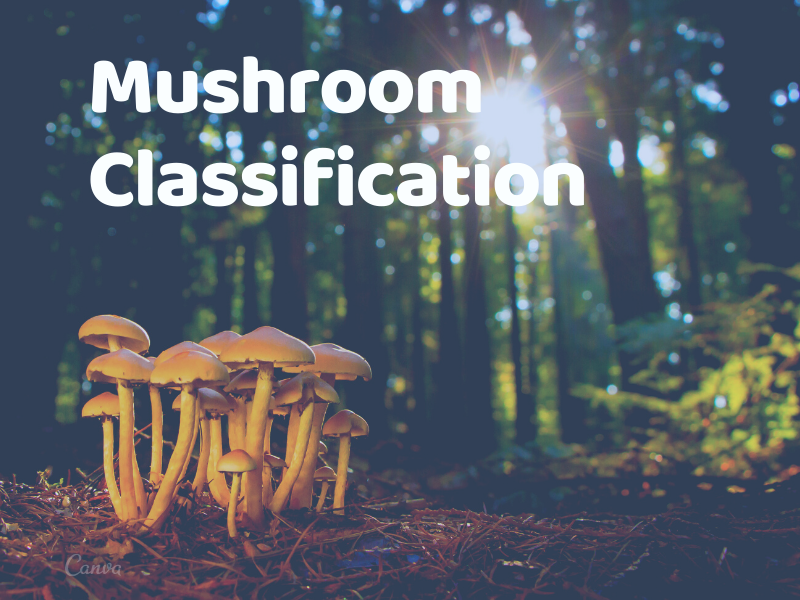
A mushroom, or toadstool, is the fleshy, spore-bearing fruiting body of a fungus, typically produced above ground on soil or on its food source. Problem Statement or Business Problem In this project, looking at the various properties of a mushroom, we will predict whether the mushroom is edible or poisonous. Attribute Information or Dataset Details: To be more understandable, let's write properties one by one. classes: edible=e, poisonous=p cap-shape: bell=b,conical=c,convex=x,flat=f, knobbed=k,sunken=s cap-surface: fibrous=f,grooves=g,scaly=y,smooth=s cap-color: brown=n, buff=b, cinnamon=c, gray=g,green=r, pink=p, purple=u, red=e,white=w,yellow=y bruises: bruises=t,no=f odor: almond=a, anise=l, creosote=c, fishy=y, foul=f, musty=m, none=n, pungent=p,spicy=s gill-attachment: attached=a,descending=d,free=f,notched=n gill-spacing: close=c,crowded=w,distant=d gill-size: broad=b,narrow=n gill-color: black=k,brown=n,buff=b,chocolate=h,gray=g,…
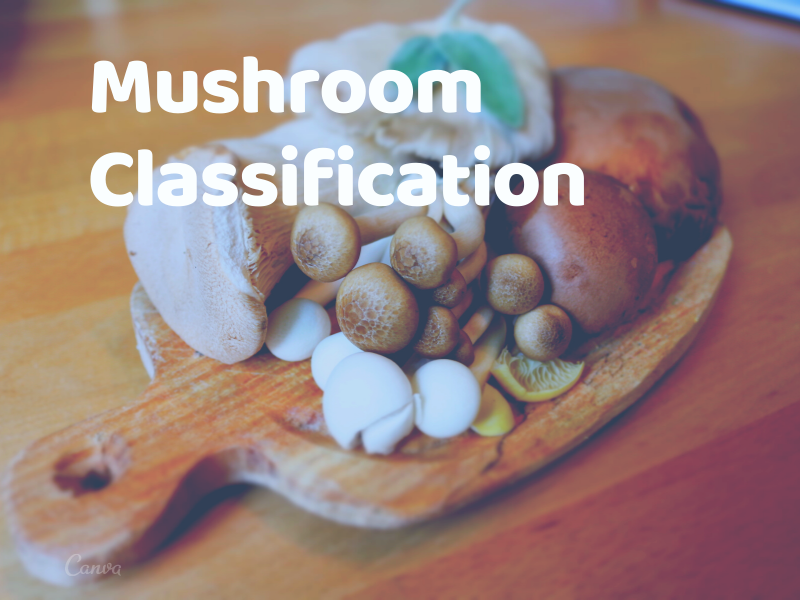
Collecting all String Columns into an Array %scala var StringfeatureCol = Array("class", "capshape", "capsurface", "capcolor", "bruises", "odor", "gillattachment", "gillspacing", "gillsize", "gillcolor", "stalkshape", "stalkroot", "stalksurfaceabovering", "stalksurfacebelowring", "stalkcolorabovering", "stalkcolorbelowring", "veiltype", "veilcolor", "ringnumber", "ringtype", "sporeprintcolor", "population", "habitat") StringIndexer encodes a string column of labels to a column of label indices. Example of StringIndexer %scala import org.apache.spark.ml.feature.StringIndexer val df = spark.createDataFrame( Seq((0, "a"), (1, "b"), (2, "c"), (3, "a"), (4, "a"), (5, "c")) ).toDF("id", "category") df.show() val indexer = new StringIndexer() .setInputCol("category") .setOutputCol("categoryIndex") val indexed = indexer.fit(df).transform(df) indexed.show() Output: +---+--------+ | id|category| +---+--------+ | 0| a| | 1| b| | 2| c| | 3|…
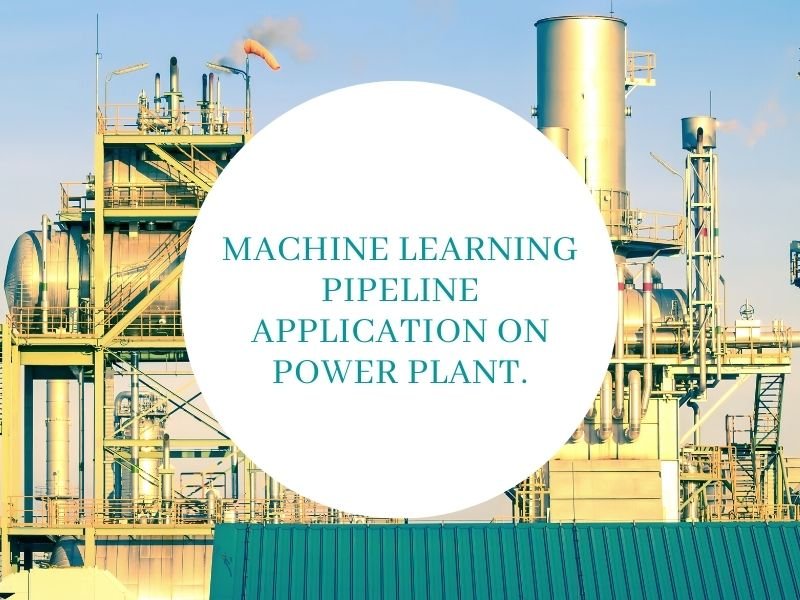
This is an end-to-end Project of performing Extract-Transform-Load and Exploratory Data Analysis on a real-world dataset, and then applying several different machine learning algorithms to solve a supervised regression problem on the dataset. Our goal is to accurately predict power output given a set of environmental readings from various sensors in a natural gas-fired power generation plant. Background Power generation is a complex process, and understanding and predicting power output is an important element in managing a plant and its connection to the power grid. The operators of a regional power grid create predictions of power demand based on historical…
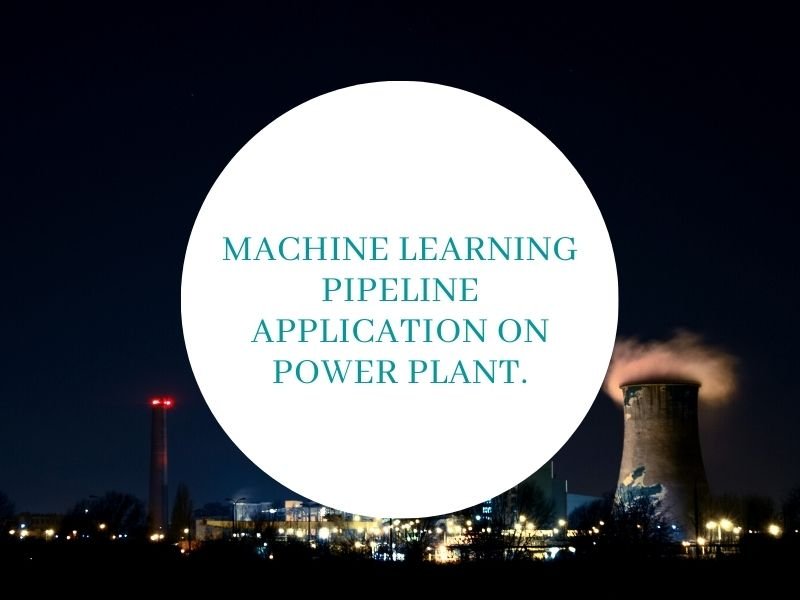
Visualize Your Data To understand our data, we will look for correlations between features and the label. This can be important when choosing a model. E.g., if features and a label are linearly correlated, a linear model like Linear Regression can do well; if the relationship is very non-linear, more complex models such as Decision Trees can be better. We can use Databrick's built in visualization to view each of our predictors in relation to the label column as a scatter plot to see the correlation between the predictors and the label. Exploratory Data Analysis (EDA) is an approach/philosophy for…